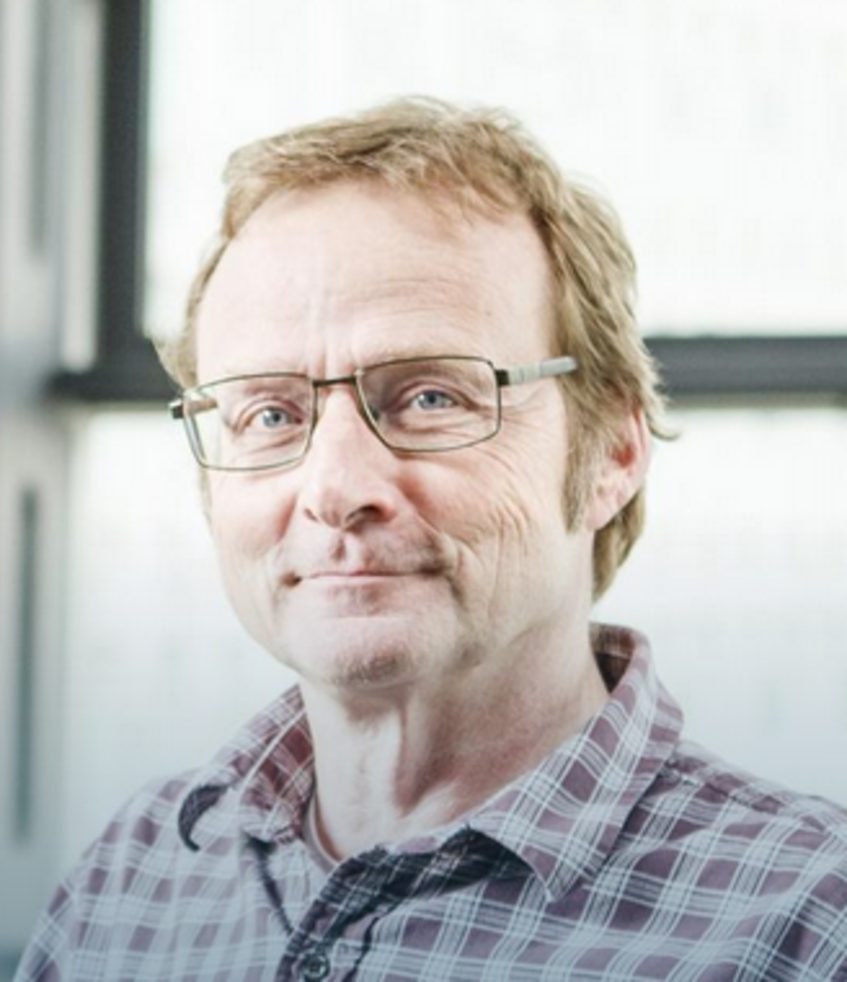
Arndt von Haeseler
Alumni
The von Haeseler lab was a key member of our consortium during the first funding phase. Arndt retired at the end of the first funding period, and we wish him the best in the next chapter of his life.
During the first funding period, the von Haeseler group collaborated extensively within our consortium and provided invaluable assistance in developing new approaches to our single-cell data.
Subproject 11: Quantitative models in stem cell-based-omics approaches
Our work consisted of mainly two research components with three specific aims. The first aim focused on establishing state-of-the-art bioinformatics workflows for production line analysis. We modified our original goals to accommodate new methods and analysis requirements from the participating groups. Additional PhD students were recruited to provide bioinformatics support, and collaboration within the consortium led to significant advancements and publications in the field of behavioral rhythms and pluripotent cell lines.
The second aim offered an improved statistical perspective on single-cell RNA sequencing (scRNA-Seq) to compute unbiased and stable gene expression profiles. We addressed the limitations of scRNA-Seq in capturing a small fraction of total mRNA content and detecting a limited number of expressed genes. We propose the Good-Turing estimator as a better alternative for library size normalization, improving the estimation of relative gene expression vectors. Simulations and collaborations with other labs validated the effectiveness of this approach, resulting in the development of an R-package called GTestimate.
The third aim, focused on single-cell RNA sequencing and phylogenetics, faced delays due to slower progress and a change in personnel. However, recent efforts have shown promise in evaluating phylogenetic approaches to understand differentiation processes using scRNA data. A technique to reduce noise in expression profiles was developed, enabling the application of phylogenomic analysis. The team plans to continue exploring this approach in the future.
Overall, the project made significant contributions through collaborations, publications, and advancements in bioinformatics workflows, statistical analysis of scRNA-Seq data, and the integration of phylogenetic approaches for understanding cellular differentiation processes.
Recent publications
Gentrius: Generating Trees Compatible With a Set of Unrooted Subtrees and its Application to Phylogenetic Terraces
Chernomor O, Elgert C, von Haeseler A. Mol Biol Evol. 2024 Nov 1;41(11):msae219. doi: 10. 1093/molbev/msae219. PMID: 39431557; PMCID: PMC11536181.
A novel approach for in vivo DNA footprinting using short double-stranded cell-free DNA from plasma
Müller J, Hartwig C, Sonntag M, Bitzer L, Adelmann C, Vainshtein Y, Glanz K, Decker SO, Brenner T, Weber GF, von Haeseler A, Sohn K. Genome Res. 2024 Sep 20;34(8):1185-1195. doi: 10. 1101/gr. 279326. 124. PMID: 39271293; PMCID: PMC11444180.
Splice_sim: a nucleotide conversion-enabled RNA-seq simulation and evaluation framework
Popitsch N, Neumann T, von Haeseler A, Ameres SL. Genome Biol. 2024 Jun 25;25(1):166. doi: 10. 1186/s13059-024-03313-8. PMID: 38918865; PMCID: PMC11514792.
Cerebral organoids display dynamic clonal growth and tunable tissue replenishment
Lindenhofer D, Haendeler S, Esk C, Littleboy JB, Brunet Avalos C, Naas J, Pflug FG, van de Ven EGP, Reumann D, Baffet AD, von Haeseler A, Knoblich JA. Nat Cell Biol. 2024 May;26(5):710-718. doi: 10. 1038/s41556-024-01412-z. Epub 2024 May 7. PMID: 38714853; PMCID: PMC11098754.
Neutral competition explains the clonal composition of neural organoids
Pflug FG, Haendeler S, Esk C, Lindenhofer D, Knoblich JA, von Haeseler A. PLoS Comput Biol. 2024 Apr 22;20(4):e1012054. doi: 10. 1371/journal. pcbi. 1012054. PMID: 38648250; PMCID: PMC11065252.
Learning From an Artificial Neural Network in Phylogenetics
Leuchtenberger AF, von Haeseler A. IEEE/ACM Trans Comput Biol Bioinform. 2024 Mar- Apr;21(2):278-288. doi: 10. 1109/TCBB. 2024. 3352268. Epub 2024 Apr 3. PMID: 38198267.
Critical Growth of Cerebral Tissue in Organoids: Theory and Experiments
Kiselev EI, Pflug F, von Haeseler A. Phys Rev Lett. 2023 Oct 27;131(17):178402. doi: 10. 1103/PhysRevLett. 131. 178402. PMID: 37955473.
ModelRevelator: Fast phylogenetic model estimation via deep learning
Burgstaller-Muehlbacher S, Crotty SM, Schmidt HA, Reden F, Drucks T, von Haeseler A. Mol Phylogenet Evol. 2023 Nov;188:107905. doi: 10. 1016/j. ympev. 2023. 107905. Epub 2023 Aug 16. PMID: 37595933.
SVhound: detection of regions that harbor yet undetected structural variation
Paulin LF, Raveendran M, Harris RA, Rogers J, von Haeseler A, Sedlazeck FJ. BMC Bioinformatics. 2023 Jan 20;24(1):23. doi: 10. 1186/s12859-022-05046-6. PMID: 36670361; PMCID: PMC9854228.
Molecular archaeology of human cognitive traits
Kaczanowska J, Ganglberger F, Chernomor O, Kargl D, Galik B, Hess A, Moodley Y, von Haeseler A, Bühler K, Haubensak W. Cell Rep. 2022 Aug 30;40(9):111287. doi: 10. 1016/j. celrep. 2022. 111287. PMID: 36044840.